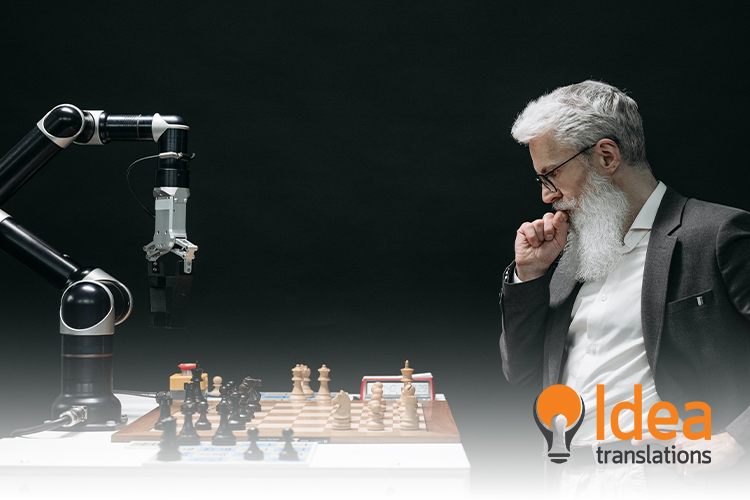
Language and artificial intelligence: the synergy of a growing relationship
When we think of artificial intelligence (AI), we usually think of computers, programming and robots. However, people with a background in linguistics are increasingly in demand in this field.
Language professionals have a lot to contribute in areas such as product development, speech recognition, search engine automation, language translation and customer service, among others. How does language strengthen the development of artificial intelligence?
Machines that evolve like the brain
The latest predictive language models can not only predict the next word as the user types, but also perform tasks that require a higher degree of comprehension, such as answering questions and summarizing documents.
When designed, these models did not seek to mimic how the human brain performs that task, MIT neuroscientists have noted that their underlying function resembles the function of the language processing centers in the human brain. “The best solutions that AI engineers have created end up being similar to the solutions found by the evolutionary process that created the human brain. Since the AI network did not seek to mimic the brain directly, it suggests that, in a sense, there has been a kind of convergent evolution between AI and nature,” says Daniel Yamins of Stanford University.
Nearly 40% of Internet users worldwide prefer to interact with chatbots rather than virtual agents. Source: Insider Intelligence
The intimate relationship between language and artificial intelligence is the subject of studies performed around the world: in several studies, linguistics collaborates with electrical engineering, computer science and cognitive science. Some of these studies explore, for example, how syntax can contribute data to machine learning, while others focus on enhancing the chatbots we use every day on websites or virtual agents, such as Siri and Alexa. There are also universities offering Ph.D. courses in linguistics and machine learning.
The future of computational linguistics
Defined as the theoretical study of language, with research methods and issues common to various languages, linguistics overlaps with other disciplines:
-Neurolinguistics.
-Psycholinguistics.
-Sociolinguistics.
-Applied linguistics.
-Computational linguistics.
-Dialectology.
-Ethnolinguistics (or Anthropological linguistics).
-Comparative linguistics.
-Historical linguistics.
-Stylistics.
Computational linguistics is also called Natural Language Processing (NLP): this is the branch of computer science (and more specifically, artificial intelligence) that deals with giving computers the ability to understand texts and spoken words in the same way as humans. NLP allows computers to process human language in the form of text or voice data.
And the future of NLP is very promising. “The conventional wisdom around AI has been that, while computers have an advantage over humans when it comes to data-driven decision making, they can’t compete on qualitative tasks. That, however, is changing. Natural language processing tools have advanced rapidly and can help with writing, coding, and discipline-specific reasoning,” they point out in a Harvard Business Review article, while warning that language-based AI will not replace certain jobs, but will automate many tasks, even for decision makers. This means that the future will be fertile ground for companies and organizations that can leverage these technologies to automate processes that are automatable, and focus their human capital on tasks that require cultural, creative and contextual knowledge. Those who see these technologies as a threat that can replace them will actually be replaced by professionals who use these tools to improve processes.
The NLP market is forecast to be nearly 14 times bigger in 2025 than in 2017: it would exceed US$43 billion by 2025. Source: Statista
Data – an increasingly important factor in the success of organizations, is ultimately text. That’s why the evolution of AI will continue to be shaped by the contribution of language. “In the future, it is possible that machine learning methods such as Knowledge Graph Completion (KGC) could make it more feasible to build linguistic resources,” point out researchers from the University of Pennsylvania and Baidu Research.
And they add some promising paths for further study and development, such as Universal Dependencies (an international project that brings databanks together with the grammars of more than 70 languages) and Contextual Embeddings (which assigns each word a representation based on its context, thus capturing the uses of words in varied contexts).
Constantly in motion, language is not only fascinating to experts, but also continues to contribute to the advancement of new technologies and, ultimately, a better quality of life for people around the world.